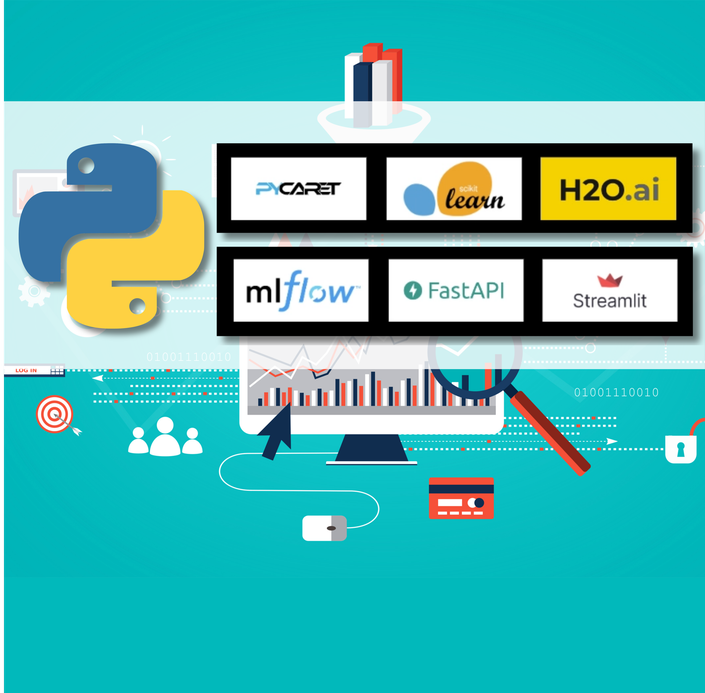
Python for Machine Learning and APIs (Course 2)
Convert a Business Problem to a Python Machine Learning API Solution.
Enroll in Course
off original price!
The coupon code you entered is expired or invalid, but the course is still available!
Here are some of the highlights of the course:
- Avoid the mistake which causes 87% of data science projects fail.
- Simulate the cost of the problem under different scenarios.
- Discover how to provide a range for how much the problem is costing the organization.
- How to connect tables to a sql database.
- Create a full blown exploratory report in 1 line of code.
- Discover how to gain insights to determine what increases the probability of customers making purchases.
- Learn how to make special engineered features to help you clean, sort, and manage data.
- Learn how to create association analysis reports to help your company discover new customer insights.
- Discover the one key feature I use to determine a 2900% increase in purchase.
- Use Pycaret to access 19 model algorithms including XGBoost, LightGBM and Catboost. (and then use Machine Learning with ALL 19 models.)
- Discover how to tune models to stabilize predictions and increase performance.
- Use my overlooked technique to select the right model for your analysis (And another method to find the most important features of your model.)
- Find out WHY a model is predicting a customer to buy.
- How to lead score customers based on probability of buying.
- Discover how to create SciKit Learn Pipelines from scratch (plus how to tune them manually using GridSearch)
- I have created a secret python code to make 100s of machine learning models in minutes (on top of that, Iβll also show you how to find the most accurate one.)
- Learn how to log advanced models like H2O (and access the AutoML leader model).
- How I created a monthly increase of $237,266 in new sales a month for a single company.
- I show you the A-Z process for creating a stand alone app which allows ANYONE to use the algorithm youβve created.
Empower non-techie people download their own Lead Scoring StrategiesBuild 100's of machine learning models in under 1 minute with this technique.
Here Are Some Screenshots From Within The Course
Avoid the mistake 90% of Data Science Teams Make

Perform Exploratory Data Analysis at the speed of light

Gain insights fast (and get quick wins for your company)

Increase your Classifier AUC 15% with these Feature Engineering Tips

Enhance your Feature Engineering Skills to uncover more insights!

Use this hack to make 19 Scikit Learn Models in under 1 minute.

Automatically sort the models by performance with only 1 line of code.

Tune your models in 1 line of code.

Discover the most important features without introducing correlation bias.

Uncover why a customer is likely to buy.

Use this trick to build scikit-learn pipelines faster

Build 100's of machine learning models in under 1 minute with this technique.

Pick the best model with this time-tested trick.

Uncover a super-power: logging in MLFlow!

Use H2O & MLFlow to build the ultimate Production-Ready Machine Learning Models.

Wow your company by sharing how your algorithm makes them $311,105 in sales every month.

Store your leader H2O Models in MLFlow and track the Machine Learning Lifecycle.

Make an App that lets your company's "non-techie" people access your business solution automatically. (And instantly become a leader in your organization)

Unlock the ultimate production tool: APIs

Make $3,600,000 in one year with your model! (and you'll likely get a promotion)

Empower non-techie people to download their own Lead Scoring Strategies

Secure your API (without involving the IT Department)

Build robust APIs in under 5 minutes

Show you're a team player by incorporating your management's input into your analysis and making a "Balanced Plan"

Get started now!
Your Instructor

Matt Dancho
Founder of Business Science and general business & finance guru, He has worked with many clients from Fortune 500 to high-octane startups! Matt loves educating data scientists on how to apply powerful tools within their organization to yield ROI. Matt doesn't rest until he gets results (literally, he doesn't sleep so don't be suprised if he responds to your email at 4AM)!
Course Curriculum
Welcome to Machine Learning APIs with Python
Available in
days
days
after you enroll
Prerequisites
Available in
days
days
after you enroll
πBonus Cheat Sheets!
Available in
days
days
after you enroll
Student Orientation (Part 1): Overview, Business Case & BSPF Framework
Available in
days
days
after you enroll
-
Startπ½ Download the Student Orientation Slides
-
Startπ₯ Student Orientation Kickstart (1:24)
-
StartWhy Learn from Me? (The Value of this Training) (1:45)
-
StartMy First BIG Project. (Story of FAILURE. And then success! The BSPF) (6:13)
-
StartThe Interview Secret. (How Students Use the BSPF to get Jobs) (2:51)
-
Start[BUSINESS CASE] How the BSPF Works (On the Course Project: Email Lead Scoring) (11:15)
Student Orientation (Part 2): Course Workflow, Software & Prerequisites
Available in
days
days
after you enroll
Course Project Setup
Available in
days
days
after you enroll
-
Startπ½ Course Project Files [File Download] (1:00)
-
StartStep 1: VSCode Setup (Building on DS4B 101-P) (3:59)
-
StartStep 2: VSCode Extensions: Python, Files, Themes & Icons (5:11)
-
StartStep 3: Install Anaconda (0:39)
-
Start***Windows Users*** Do this! (Before Using Conda via VSCode Terminal)
-
StartOption 1: How Jonathan Regenstein got his python environment up in 5 minutes
-
StartOption 2: (20 Minute Experimental Installation): Minimal Python Environment (6:31)
-
StartOption 3: (Fully Reproducible Installation) Step 4: Environment Setup with Conda (6:05)
-
StartOption 3: (Fully Reproducible Installation) Step 5: Add Python Packages from Requirements.txt (4:16)
-
StartPython Environment Wrapup (2:03)
Module 0: Jumpstart with ML & APIs
Available in
days
days
after you enroll
Jumpstart Part 1: Intro To Machine Learning With Pycaret
Available in
days
days
after you enroll
-
StartReading Data (SQL Database Connection) (4:05)
-
StartExamining Email Subscribers & Transactions Tables (5:26)
-
StartSimplified Data Preparation (5:36)
-
StartPycaret: Classifier Setup (5:28)
-
StartPycaret: Make an XGBoost Model (4:02)
-
StartPycaret: Lead Scoring (Prediction) (5:16)
-
StartPycaret: Save & Load ML Models (3:40)
-
StartConclusions (Pycaret) (3:52)
Jumpstart Part 2: Intro To APIs with Fast API
Available in
days
days
after you enroll
β
Code Checkpoint: Module 0 - Jumpstart
Available in
days
days
after you enroll
π₯³ UNANNOUNCED BONUS: Jumpstart Part 3: Streamlit Jumpstart
Available in
days
days
after you enroll
-
Startπ½ Streamlit Introduction [Download the Streamlit Starter Files] (1:07)
-
Startπ Streamlit Documentation (2:29)
-
StartHow to Run Your Streamlit App (3:49)
-
StartCustomizing the Theme Appearance (1:09)
-
Startst.write(): Write arguments to the app (2:14)
-
Startst.file_uploader(): Uploading Data (2:51)
-
Startst.write(): Displaying Data (2:16)
-
Startπ Chart 1: Sales Over Time (5:26)
-
Startπ₯§ Chart 2: Customer Segment by Region (3:10)
-
Startπ Chart 3: Product Analysis (4:32)
-
StartUser Input: Form Feedback (2:36)
-
StartAdd a Footer to Your App (1:27)
-
StartConclusions: Streamlit is Awesome! (0:30)
-
Startπ½ Code Checkpoint [File Download]
Module 1: Business Understanding
Available in
days
days
after you enroll
1.1 Initial Cost Analysis: Quick Rough-Cut Costs
Available in
days
days
after you enroll
1.2 Cost Table: Modeling Time-Varying Costs π
Available in
days
days
after you enroll
-
StartUnderstanding Growth Rates (3:33)
-
StartCost Table: No Growth Scenario, Part 1 (5:10)
-
StartCost Table: No Growth Scenario, Part 2 (3:58)
-
StartCost Table: Compounding Growth Scenario, Part 1 (5:07)
-
StartCost Table: Compounding Growth Scenario, Part 2 (4:39)
-
StartFIX 1: Lost Customers Calculation
-
Startπ Understanding Cost: Comparing Growth vs No-Growth Scenarios (2:38)
-
Startπ€Is Reducing the Problem Worth It? (2:49)
1.3 "Parameter-izing" Your Cost Analysis (with Functions)
Available in
days
days
after you enroll
1.4 Cost Simulation
Available in
days
days
after you enroll
-
StartAre the Objectives Being Met? (The Importance of Simulation) (3:06)
-
StartParameter Grid: Cartesian Product with Janitor expand_grid() (6:05)
-
StartβοΈRun the Simulation (List Comprehension) (8:00)
-
StartFunction: Simulate Unsubscriber Costs, Part 1 (4:09)
-
StartFunction: Simulate Unsubscriber Costs, Part 2 (3:06)
-
StartFunction: Simulation Unsubscriber Costs, Part 3 (2:32)
1.5 Heat Map: Cost Simulation Visualization
Available in
days
days
after you enroll
1.6 Email Lead Scoring Package: The Cost Calculations Module
Available in
days
days
after you enroll
-
StartEmail Lead Scoring Package: Introduction (0:35)
-
StartSetting Up the Email Lead Scoring Package (2:45)
-
StartAdding the Cost Table Function (4:50)
-
StartFix 1: Correcting Lost Customers Calculation
-
Startπ Importing functions into __init__.py (5:11)
-
StartAdding the Total Cost Function (3:17)
-
StartπPandas Flavor: Method Chaining (4:09)
-
StartAdding the Simulation Function (5:18)
-
StartAdding the Plot Simulation (Heat Map) Function (5:19)
β
Code Checkpoint: Module 1 - Business Understanding
Available in
days
days
after you enroll
Module 2 - Data Understanding & KPIs
Available in
days
days
after you enroll
2.1 Data Understanding - Collecting Data & Discovering What It Means
Available in
days
days
after you enroll
-
StartData Understanding: Python Libraries & ELS Module Import (3:04)
-
StartSQL Database Connection (4:41)
-
StartThe Products Table (4:25)
-
StartThe Subscribers Table (7:07)
-
StartThe Tags Table (4:15)
-
StartThe Transactions Table (5:05)
-
StartThe Website Table (4:29)
-
StartClose the Connection (2:00)
2.2 Combining & Analyzing The Data
Available in
days
days
after you enroll
-
StartCombining The Data (Our Data Strategy) (1:42)
-
StartAdding the Target (Made a Purchase) (3:40)
-
StartAnalysis: Who is Purchasing? (2:16)
-
StartGeographic Analysis Part 1 (5:45)
-
StartGeographic Analysis Part 2 (2:49)
-
StartTop N Countries (3:42)
-
StartUsing Quantile To Understand Differences In Group (1:45)
-
StartTag Analysis Part 1 (2:23)
-
StartTag Analysis Part 2 (4:58)
-
StartTag Analysis Part 3 (2:57)
π¬ 2.3 Sweetviz & KPIs
Available in
days
days
after you enroll
2.4 Data Import Functions (Add to the email_lead_scoring module)
Available in
days
days
after you enroll
-
StartDatabase Connection: db_read_els_data() (5:41)
-
StartSubscribers: db_read_els_data() (2:20)
-
StartTags: db_read_els_data() (2:41)
-
StartTarget: db_read_els_data() (3:26)
-
StartTable Names: db_read_els_table_names() (2:19)
-
StartRaw Tables: db_read_raw_els_table() (3:44)
-
Startdatabase.py (6:25)
-
StartTest Your Module Out (3:44)
2.5 Exploratory Functions (Add to the email_lead_scoring module)
Available in
days
days
after you enroll
β
Code Checkpoint: Module 2 - Data Understanding & KPIs
Available in
days
days
after you enroll
β°οΈ CHALLENGE #1 - Who Has Purchased Specific Products?
Available in
days
days
after you enroll
Module 3 - Data Processing Pipelines
Available in
days
days
after you enroll
3.1 Feature Exploration
Available in
days
days
after you enroll
3.2 Feature Engineering & Data Preprocessing
Available in
days
days
after you enroll
-
StartDate Features (Time On Email List) (4:41)
-
StartText Features (Email Provider) (2:47)
-
StartRate Features (Activity Per Time) (2:57)
-
StartOne-To-Many Features (Tags) (5:18)
-
StartMerging One-To-Many Features (Tags) (5:01)
-
StartFill Missing One-To-Many Features Using Regex (Tags) (6:26)
-
StartReducing High Cardinality Features (Country Code) (6:45)
π¬ 3.3 Sweetviz Report (Round 2) - Analyzing Engineered Features
Available in
days
days
after you enroll
3.4 Data Processing Pipeline Functions
Available in
days
days
after you enroll
-
StartLibraries Needed (1:13)
-
StartCreate The Data Processing Pipeline Function (2:11)
-
StartAdd Date Features (1:08)
-
StartAdd Email Features (1:52)
-
StartAdd Activity Features (2:36)
-
StartFill NA Regex (0:51)
-
StartAdjust High Cardinality Features (1:14)
-
StartImproving The Data Pipeline Function (3:25)
-
StartTry Out Our ELS Package (5:42)
β
Code Checkpoint: Module 3 - Data Processing Pipelines
Available in
days
days
after you enroll
Module 4 - Machine Learning with Pycaret (Deep Dive)
Available in
days
days
after you enroll
4.1 Preparing For Pycaret Classifier Setup (Don't mess this up, garbage in = garbage out)
Available in
days
days
after you enroll
4.2 Pycaret Classifier Setup: clf.setup()
Available in
days
days
after you enroll
4.3 Machine Learning With Pycaret's Compare Models
Available in
days
days
after you enroll
4.4 Plotting the Model Performance (Model Selection)
Available in
days
days
after you enroll
4.5 Individual Models: Making and Hyperparameter Tuning
Available in
days
days
after you enroll
4.6 Explainable AI: Model Interpretability
Available in
days
days
after you enroll
4.7 Ensembles (Blending), Calibrating, and Finalizing Classification Models
Available in
days
days
after you enroll
4.8 Predictions & Lead Scoring (And Model Storage)
Available in
days
days
after you enroll
4.9 Lead Scoring Function (Adding Into Your Email Lead Scoring Module)
Available in
days
days
after you enroll
β
Code Checkpoint: Module 4 - Machine Learning with Pycaret
Available in
days
days
after you enroll
Module 5: Advanced Machine Learning with Scikit Learn & H2O
Available in
days
days
after you enroll
π§ 5.1 Scikit Learn Pipelines
Available in
days
days
after you enroll
-
StartScikit Learn Pipelines: Libraries & Common Functions (3:39)
-
StartLoad a Pycaret Model (2:31)
-
StartWhat is a Pycaret Model? (4:44)
-
StartData Setup: X and y (5:27)
-
StartTrain / Test Split (3:16)
-
StartCreating A Scikit Learn Pipeline, Part 1: Column Transformers (3:51)
-
StartFIX: Remainder Passthrough
-
StartCreating A Scikit Learn Pipeline, Part 2: Pipelines (1:52)
-
StartCreating A Scikit Learn Pipeline, Part 3: Fit & Predict (2:05)
-
StartCreating A Scikit Learn Pipeline, Part 4: Metrics (7:49)
-
StartHyperparameter Tuning in Scikit Learn, Part 1: GridsearchCV() (6:01)
-
StartHyperparameter Tuning in Scikit Learn, Part 2: Fitting, Best Model Parameters (1:59)
-
StartHyperparameter Tuning in Scikit Learn, Part 3: Metrics (4:08)
-
StartPycaret vs Scikit Learn (3:38)
-
StartJoblib for Saving / Loading Scikit Learn Models (1:41)
-
StartConclusions (1:44)
π 5.2 H2O Automated Machine Learning
Available in
days
days
after you enroll
-
StartH2O Overview & Setup (2:25)
-
StartH2O Initialization: h2o.init() (3:20)
-
StartH2OFrame Part 1: h2o.H2OFrame() and h2o.describe() (2:00)
-
StartH2OFrame Part 2: Setting the Target Column as ENUM with h2o.asfactor() (1:53)
-
StartSpecifying Predictor (x) and Target (y) Columns (3:41)
-
StartH2O AutoML Part 1: H2OAutoML() (4:08)
-
StartH2O AutoML Part 2: Training the AutoML (4:21)
-
StartSaving & Loading H2O Models (4:21)
-
StartPredictions & Lead Scoring with H2O (3:11)
-
StartConclusions (1:46)
β
Code Checkpoint - Module 5: Advanced Machine Learning
Available in
days
days
after you enroll
Module 6: MLFlow
Available in
days
days
after you enroll
6.1 MLFlow + Pycaret: Interfacing with MLFlow Experiments API
Available in
days
days
after you enroll
-
StartGetting Started (0:58)
-
StartPycaret's MLFlow Integration (3:44)
-
StartMLFlow User Interface (UI) (8:05)
-
StartMLFlow Tracking API (1:19)
-
StartExperiments Part 1 (Listing Experiments) (2:14)
-
StartExperiments Part 2 (Programmatic Use & Attributes) (2:07)
-
StartSearching Experiments & Runs (7:15)
-
StartTime Saver: Pycaret clf.get_logs() (1:35)
-
StartWorking With Runs (3:17)
-
StartPredictions Part 1: Default Predictions (2:15)
-
StartProblem: Need Lead Scores (2 Solutions) (4:37)
-
StartConclusions (2:35)
6.2 MLFlow + H2O (And Scikit Learn): Using the Tracking API
Available in
days
days
after you enroll
-
StartH2O + MLFlow Integration (1:32)
-
StartH2O Setup (Recap from Module 5) (2:42)
-
StartMLFlow Tracking Setup (6:49)
-
StartMLFlow UI (0:41)
-
StartStarting an MLFlow Run (0:58)
-
StartH2O AutoML (Recap from Module 5) (2:36)
-
StartLogging Models (H2O & Scikit Learn) (2:48)
-
StartLogging Metrics (2:02)
-
StartSetting Tags (2:50)
-
StartModel URI Location (1:08)
-
StartStoring Custom Artifacts Part 1: Getting the Full Leaderboard (Extra Cols) (2:28)
-
StartStoring Custom Artifacts Part 2: Logging the Leaderboard as a CSV File (5:46)
-
StartEnding an MLFlow Run (0:46)
-
StartMaking Predictions from MLFlow H2O Models (2:16)
-
StartConclusions (1:06)
6.3 Creating Custom MLFlow Functions (for Email Lead Scoring Module)
Available in
days
days
after you enroll
-
StartAdding MLFlow Functionality to our Email Lead Scoring (ELS) Module (6:42)
-
StartMaking the Get Best Run Function (5:38)
-
StartPredict with the MLFlow Model (6:50)
-
StartMaking the MLFlow Lead Scoring Function (7:36)
-
StartLet's Add our Functions to the ELS Module (7:14)
-
StartTesting the MLFlow Lead Scoring Workflow (3:41)
β
Code Checkpoint - Module 6: MLFlow
Available in
days
days
after you enroll
Challenge 2: Make a Scikit Learn + MLFlow Tracked Lead Scoring Model
Available in
days
days
after you enroll
Module 7: Return On Investment (ROI)
Available in
days
days
after you enroll
7.1 Cost vs Savings Tradeoff
Available in
days
days
after you enroll
-
StartIntroduction to Cost vs Savings Tradeoff (5:32)
-
StartLead Targeting Strategy (6:28)
-
StartThreshold Selection (4:20)
-
StartAggregating the Results (3:21)
-
StartConfusion Matrix Calculations (Part 1): Try-Except (6:53)
-
StartConfusion Matrix Calculations (Part 2): Summaries (2:24)
-
StartPreliminary Expected Value Calcs (Part 1): Savings Cold that are Not Targeted (3:55)
-
StartPreliminary Expected Value Calcs (Part 2): Cost Missed Sales that are Not Targeted (2:03)
-
StartPreliminary Expected Value Calcs (Part 3): Cost Hot Leads Targeted that Unsubscribe (2:18)
-
StartPreliminary Expected Value Calcs (Part 4): Savings Made Purchases (1:52)
-
StartExpected Value (Part 1): 4 Important Lead Strategy Values (4:51)
-
StartExpected Value (Part 2): Summary Output for Management (3:08)
-
StartConclusions: ROI Part 1 (1:46)
7.2 Threshold Optimization & Profit Maximization
Available in
days
days
after you enroll
-
StartIntroduction to Threshold Optimization (2:20)
-
StartFunction 1: Making the Lead Strategy Function (from the Scored Leads) (7:08)
-
StartFunction 1: Lead Strategy (Functional Workflow) (2:54)
-
StartFunction 2: Aggregating the Lead Strategy Results (2:13)
-
StartFunction 2: Aggregating the Lead Strategy (Functional Workflow) (2:06)
-
StartFunction 3: Expected Value Calculation (8:21)
-
StartFunction 3: Expected Value Calculation (Functional Workflow) (5:38)
-
StartFunction 4: Create the Threshold Table (Part 1: Setting up the function args) (5:36)
-
StartFunction 4: Create the Threshold Table (Part 2: List Comprehension as a Loop) (9:33)
-
StartFunction 4: Create the Threshold Table (Part 3: Highlighting Max Values) (3:53)
-
StartFunction 4: Create the Threshold Table (Functional Workflow) (3:33)
-
StartFunction 5: Select the Best Threshold (Part 1: The 2 Criteria) (3:46)
-
StartFunction 5: Select the Best Threshold (Part 2: The 1st Filter Mask) (5:10)
-
StartFunction 5: Select the Best Threshold (Part 3: The 2nd Filter Mask) (6:17)
-
StartFunction 5: Select the Best Threshold (Functional Workflow) (2:18)
-
StartFunction 6: Get Expected Value (3:56)
-
StartFunction 6: Get Expected Value (Functional Workflow) (1:56)
-
StartFunction 7: Plot the Optimal Threshold (7:10)
-
StartFunction 7: Plot the Optimal Threshold (Functional Workflow) (1:40)
-
StartFunction 8: Optimal Strategy (Part 1: Function Setup) (3:25)
-
StartFunction 8: Optimal Strategy (Part 2: Thresh Table) (3:49)
-
StartFunction 8: Optimal Strategy (Part 3: Optimal Thresh & Expected Value) (3:22)
-
StartFunction 8: Optimal Strategy (Part 4: Thresh Plot & Final Lead Strategy) (3:46)
-
StartFunction 8: Optimal Strategy (Functional Workflow) (3:33)
-
StartConclusions: ROI Part 2 (3:13)
7.3 Creating Lead Strategy Automation Functions (for Email Lead Scoring Module)
Available in
days
days
after you enroll
β
Code Checkpoint - Module 7: ROI
Available in
days
days
after you enroll
π¨ Module 8: FastAPI (Deep-Dive)
Available in
days
days
after you enroll
8.1 Lead Scoring API with FastAPI
Available in
days
days
after you enroll
-
StartWelcome to Part 1: Creating a FastAPI to Lead Score (3:25)
-
StartIntroduce Your API: Adding a Home Screen at Your API's Webpage Root (5:16)
-
StartRun the API: Fire up the API and inspect it's Documentation (2:49)
-
StartEndpoint 1: GET /get_email_subscribers - Used to get data from your API (5:12)
-
StartTesting Our API Endpoint 1: GET /get_email_subscribers (4:54)
-
StartEndpoint 2: POST /data - Used to demonstrate how to send and retrieve data from an API (8:51)
-
StartEndpoint 3: POST / predict - Used to demonstrate how to run an ML model and return predictions (8:04)
-
StartEndpoint 4 (Part 1): POST /calculate_lead_strategy (5:16)
-
StartEndpoint 4 (Part 2): POST /calculate_lead_strategy (3:43)
-
StartEndpoint 4 (Part 3): POST /calculate_lead_strategy (3:24)
-
StartEndpoint 4 (Part 4): POST /calculate_lead_strategy (2:48)
-
StartEndpoint 4 (Part 5): POST /calculate_lead_strategy (4:09)
-
StartEndpoint 4 (Part 6): POST /calculate_lead_strategy (1:55)
8.2 API Security (API Keys)
Available in
days
days
after you enroll
β
Code Checkpoint - Module 8: FastAPI
Available in
days
days
after you enroll
Module 9: Streamlit App
Available in
days
days
after you enroll
9.1 Streamlit Jumpstart
Available in
days
days
after you enroll
-
StartStreamlit Introduction (1:07)
-
Startπ Streamlit Documentation (2:29)
-
StartHow to Run Your Streamlit App (3:49)
-
StartCustomizing the Theme Appearance (1:09)
-
Startst.write(): Write arguments to the app (2:14)
-
Startst.file_uploader(): Uploading Data (2:51)
-
Startst.write(): Displaying Data (2:16)
-
StartChart 1: Sales Over Time (5:26)
-
StartChart 2: Customer Segment by Region (3:10)
-
StartChart 3: Product Analysis (4:32)
-
StartUser Input: Form Feedback (2:36)
-
StartAdd a Footer to Your App (1:27)
-
StartConclusions: Streamlit is Awesome! (0:30)
9.2 Lead Scoring Application (Front End)
Available in
days
days
after you enroll
-
StartTransitioning from the Streamlit Jumpstart (0:38)
-
StartSetting Up for the Lead Scoring App (7:00)
-
StartUpload and Cache Large Data (3:24)
-
StartDisplay the Leads Data (3:16)
-
StartUser Inputs: Used to Perform the Lead Scoring Analysis (7:26)
-
StartConnecting to the API and Making Requests (6:44)
-
StartCollect the JSON Response and Get the Analysis Results (5:01)
-
StartDisplay the Analysis Results: Lead Strategy Summary (Expected Savings), Threshold Plot, and Sample Strategy (4:55)
-
StartDownload the Lead Strategy Results as a CSV File (3:33)
-
StartTidy Our Workspace Up (0:24)
9.3 App Security: Adding Login Authentication
Available in
days
days
after you enroll
β
Code Checkpoint - Module 9: Streamlit App
Available in
days
days
after you enroll
π Congratulations! You Did It! Get Your Course Certificate!
Available in
days
days
after you enroll
Frequently Asked Questions
When does the course start and finish?
The course starts now and never ends! It is a completely self-paced online course - you decide when you start and when you finish.
How long do I have access to the course?
How does lifetime access sound? After enrolling, you have unlimited access to this course for as long as you like - across any and all devices you own.
What if I am unhappy with the course?
We would never want you to be unhappy! If you are unsatisfied with your purchase, contact us in the first 30 days and we will give you a full refund.
off original price!
The coupon code you entered is expired or invalid, but the course is still available!